As somebody who’s passionate concerning the transformative energy of know-how, it’s fascinating to see clever computing – in all its numerous guises – bridge the schism between fantasy and actuality. Organisations the world over are within the course of of creating the place and the way these developments can add worth and edge them nearer to their objectives. The thrill is palpable.
Nevertheless, it is crucial that this pleasure doesn’t blind us to the risks, propelling us forward with out having taken the proper preparatory steps or with out understanding the challenges that shall be encountered alongside the best way.
Getting ready for a synthetic intelligence (AI)-fueled future, one the place we are able to benefit from the clear advantages the know-how brings whereas additionally the mitigating dangers, requires multiple article. This primary article emphasizes information because the ‘foundation-stone’ of AI-based initiatives.
Establishing a Knowledge Basis
The shift away from ‘Software program 1.0’ the place functions have been primarily based on hard-coded guidelines has begun and the ‘Software program 2.0’ period is upon us. Software program improvement, as soon as solely the area of human programmers, is now more and more the by-product of knowledge being rigorously chosen, ingested, and analysed by machine studying (ML) programs in a recurrent cycle. On this new period the function of people within the improvement course of additionally modifications as they morph from being software program programmers to turning into ‘information producers’ and ‘information curators’ – tasked with making certain the standard of the enter.
This might be easy activity have been it not for the truth that, through the digital-era, there was an explosion of knowledge – collected and saved all over the place – a lot of it poorly ruled, ill-understood, and irrelevant. Knowledge lakes have been amassed throughout a time when organisations have been pre-occupied with ‘infrastructure-first transformation’ initiatives. And, whereas it might be helpful to digitize enterprise processes, unburden your self from siloed multi-generational IT, and drive cloud-first mandates, it would solely get you to date on the transformation continuum.
Knowledge Centricity
Ahead-thinking transformation leaders have realised that extra focus must be positioned on ‘data-centric worth creation’ and have made this the pre-eminent organising precept of their organisations. “Knowledge-first,” as a foundation for know-how and different vital funding choices, can:
- Spur new working fashions that assist them differentiate and develop
- Create ‘hyper-personalised’ digital moments and experiences that drive loyalty
- Enhance foresight and increase predictive capabilities
These leaders are doing so not simply to assist them totally embrace the digital ‘now,’ however to organize for and capitalise on the AI-fuelled digital ‘subsequent.’
Exposing the Blindspot
There may be little doubt that the subsequent wave of know-how, pushed by better automation and computational intelligence, will depend on information greater than any previous period. To take full benefit of those developments information have to be:
- Nicely understood and effectively organised
- Frequently analysed for relevance and cleansed
- Sensibly situated the place it will possibly add most worth and be accessed in a frictionless, cost-effective manner
- Rigorously chosen to drive the optimum enterprise outcomes
- Tightly ruled and controlled such that it’s compliant and ethically sound
To miss or downplay the significance of any of those concerns is to doubtlessly construct your AI future on pillars of sand.
There may be proof to recommend that there’s a blind spot relating to information within the AI context. Many organisations focus too closely on high quality tuning their computational fashions of their pursuit of ‘quick-wins.’ Nevertheless, opposite to fashionable perception, AI success just isn’t about tweaking and recalibrating fashions, it’s about tweaking information, regularly.
As soon as constructed, the computational fashions ought to stay comparatively static. Most trade specialists imagine it’s information availability, high quality, and understanding which are the most important determinants of success in AI. With out them an organisations’ AI exploits carry important danger, notably because of the triple-threats of knowledge bias, mis-labelling, and poor choice.
Regardless of soundings on this from main thinkers corresponding to Andrew Ng, the AI neighborhood stays largely oblivious to the essential information administration capabilities, practices, and – importantly – the instruments that make sure the success of AI improvement and deployment.
Addressing the Problem
Knowledge-centric AI is evolving, and may embody related information administration disciplines, strategies, and abilities, corresponding to information high quality, information integration, and information governance, that are foundational capabilities for scaling AI. Additional, information administration actions don’t finish as soon as the AI mannequin has been developed. To assist this, and to permit for malleability within the ways in which information is managed, HPE has launched a brand new initiative referred to as Dataspaces, a strong cloud-agnostic digital providers platform aimed toward placing extra management into the arms of knowledge producers and curators as they construct clever programs.
Addressing, head on, the information gravity and compliance concerns that exist for vital datasets, Dataspaces offers information producers and customers frictionless entry to the information they want, after they want it, supporting higher integration, discovery, and entry, enhanced collaboration, and improved governance as well.
Because of this organisations can lastly leverage an ecosystem of AI-centric information administration instruments that mix each conventional and new capabilities to organize the enterprise for achievement within the period of choice intelligence. An incredible instance of that is Novartis.
Suggestions for Knowledge and AI Leaders
In abstract, to be able to make sure that AI packages are a hit from the outset, organisations ought to take the next data-related steps:
- Formalise each ‘data-centric AI’ and ‘AI-centric information’ as a part of information administration technique with metadata and information material as key foundational parts.
- Set coverage guardrails that embody necessary minimums about ‘information health’ for AI, to guard in opposition to bias, mislabelling, or irrelevance.
- Outline the suitable codecs, instruments, and metrics for AI-centric information as early as doable, stopping the necessity to reconcile a number of information approaches as AI scales.
- Search variety of knowledge, algorithms, and folks inside the AI provide chain to make sure worth is realised and moral approaches are taken.
- Set up roles and obligations to handle information in assist of AI, leveraging AI engineering and information administration experience (inside and exterior) and approaches to assist ongoing deployment and manufacturing makes use of of AI.
The following article will concentrate on how one can enhance the transparency and ‘explainability’ of AI programs to be able to successfully take away bias inside the information or the computational fashions – decreasing the inherent danger within the course of.
To study extra, go to HPE.
____________________________________
About Andrew P. Ayres MBA
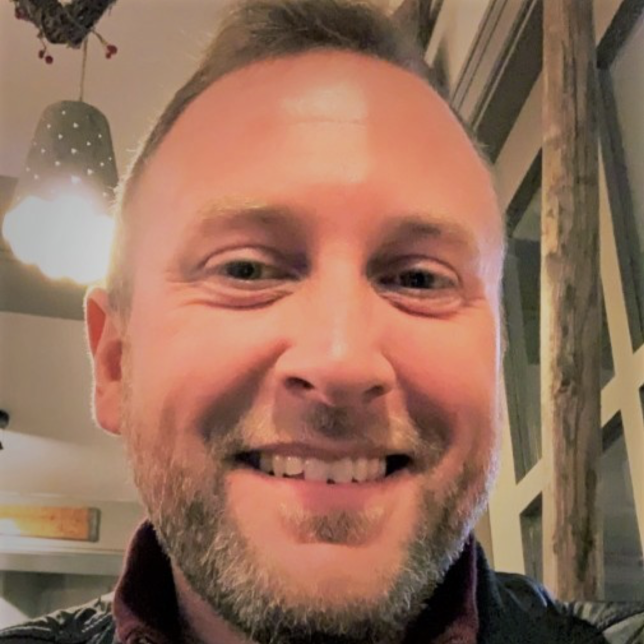